Two papers and invited sessions @ virtual CDC 2021
- conferences
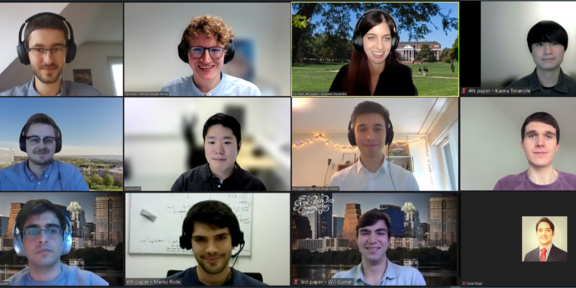
Nils presented a new scheme to evaluate piecewise affine control laws in an encrypted fashion. Contrary to existing approaches, the novel setup focuses on two-party protocols. The key insight is that a tailored max-out neural network approximation of the control law is well-suited for an evaluation via additive secret sharing and garbled circuits. This greatly contributes to the efficiency and opens new opportunities for applying encrypted control. Contact Nils for further details or have a look at the paper:
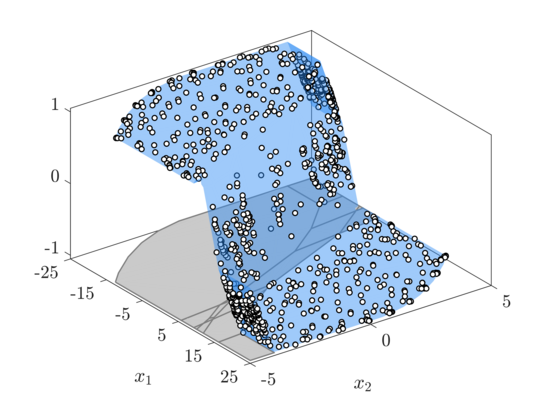
K. Tjell, N. Schlüter, P. Binfet, and M. Schulze Darup, Secure learning-based MPC via garbled circuit, 60th IEEE Conference on Decision and Control (CDC), 2021. Preprint: arXiv:2112.03654
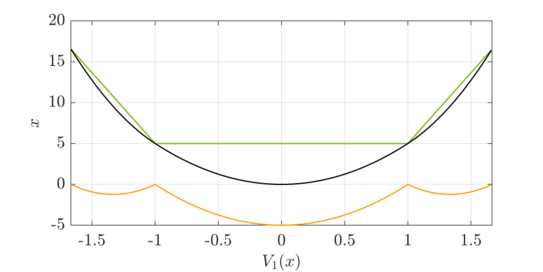
Dieter's talk focused on tailored neural networks for model predictive control (MPC). In the corresponding paper, he investigated suitable topologies for the approximation of an MPC law, the corresponding optimal value function, or the Q-function. A central observation is that it is always possible to find a topology which allows an exact representation of a piecewise quadratic optimal value function or Q-function for one-dimensional states (see picture 2). This can be exploited for more efficient learning and controller evaluation. Contact Dieter for further details or have a look at the paper:
D. Teichrib and M. Schulze Darup, Tailored neural networks for learning optimal value functions in MPC, 60th IEEE Conference on Decision and Control (CDC), 2021. Preprint: arXiv:2112.03975