Goals and Directions
Control systems are getting more and more complex and solving control tasks increasingly relies on interdisciplinary approaches combining elements from mechatronics and cybernetics. Against this background, we aim for tailored control schemes that address the special needs of such cyberphysical systems. In particular, we design networked and autonomous control systems involving numerical optimization, machine learning, and cybersecurity.
Have a look at the following selection of research directions or study our projects and publications for further details!
Secure control for networked systems
Cloud-computing and distributed computing are becoming ubiquitous in modern control systems such as smart grids, building automation, robot swarms, or intelligent transportation systems. While cloud-based and distributed control schemes offer many opportunities, they also increase the risk of cyberattacks. In fact, the involved communication and evaluation of sensitive data via public networks and on thirdparty platforms promote eavesdropping and manipulation of data. Future control schemes should counteract those threats and ensure confidentiality and integrity of the involved data. We address these needs with tailored control schemes that combine methods from cryptography, numerical optimization, and control.
Related projects
Applied encrypted control for critical infrastructure (Aperitif)
Encrypted optimization-based control for networked systems (EpicNets)
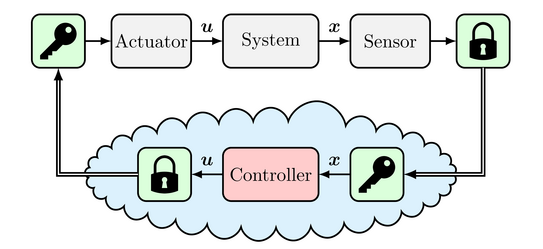
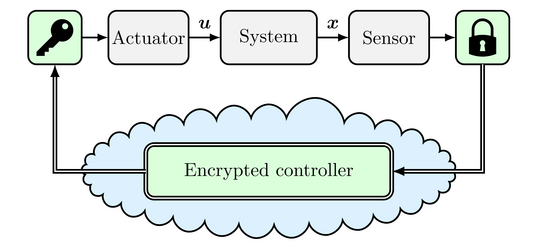
Tailored learning-based control
Details follow.
Related publications
N. Schlüter and M. Schulze Darup, Novel convex decomposition of piecewise affine functions, in Proc. of the IFAC World Congress, 2020.
M. Schulze Darup, Exact representation of piecewise affine functions via neural networks, in Proc. of the 2020 European Control Conference (ECC), pp. 1073-1078, 2020. DOI: 10.23919/ECC51009.2020.9143957
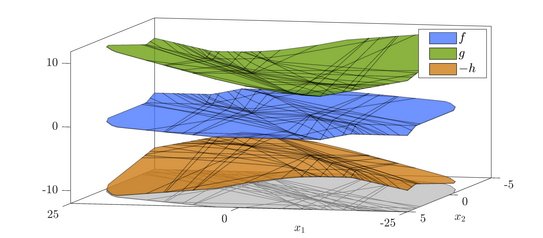
New approaches to model predictive control
Model predictive control (MPC) is one of the most powerful, widely-used, and well-studied control schemes. Its underlying idea can be traced back to the 60s. Since then, countless successful applications and theoretical improvements have been reported. Nevertheless, the theory for MPC is far from complete and novel variants and implementations pop up regurlarly. We contribute structural insights on linear and nonlinear MPC, fast real-time implementations, and secure controller evaluations.
Related publications
M. Schulze Darup, M. Klädtke, and M. Mönnigmann, Exact solution to a special class of nonlinear MPC problems, 7th IFAC Conference on Nonlinear Model Predictive Control (NMPC), 2021 (submitted).
M. Schulze Darup, G. Book, and P. Giselsson, Towards real-time ADMM for linear MPC, in Proc. of the 2019 European Control Conference, pp. 4276-4282, 2019. DOI: 10.23919/ECC.2019.8796239
M. Schulze Darup and M. Cannon, Some observations on the activity of terminal constraints in linear MPC, in Proc. of the 2016 European Control Conference, pp. 770-775, 2016. DOI: 10.1109/ECC.2016.7810382
