Paper in IEEE L-CSS
- publications
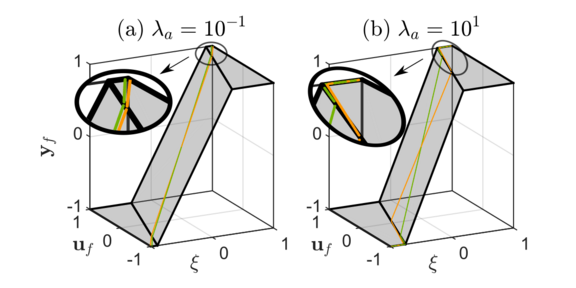
An increasingly popular data-driven predictive control (DPC) scheme, based on Willems' fundamental lemma, uses linear combinations of collected trajectory data to make predictions instead of relying on a previously estimated system model. While exact predictions are typically obtained only for LTI systems and exact data, regularized variants of this approach have shown very good performance in the presence of noise and nonlinearities.
In the paper, we introduce the concept of implicit predictors, which can be interpreted as the predictive behavior that is implicitly attributed to the data-generating system by the DPC scheme. As such, an implicit predictor acts as an implicitly estimated model, which is an opposite viewpoint to the widespread indirect vs. direct (i.e., with or without model estimation) interpretation. To demonstrate this concept, we derive implicit predictors for two popular regularization choices and discuss the influence of output constraints on the predictive behavior. Contact Manuel for further details or have a look at the paper:
M. Klädtke and M. Schulze Darup. Implicit predictors in regularized data-driven predictive control, IEEE Control Systems Letters, vol. 7, pp. 2479-2484, 2023. DOI: 10.1007/LCSYS.2023.3285104, Preprint:
arXiv:2307.10750